Investing in Maxim AI
.png?rect=231,0,978,1088&w=320&h=356&fit=min&auto=format)
Building Infrastructure of AI Adoption
.png?rect=83,0,1275,1088&w=320&h=273&fit=min&auto=format)
Investing in Maxim AI
.png?rect=231,0,978,1088&w=320&h=356&fit=min&auto=format)
Building Infrastructure of AI Adoption
.png?rect=0,159,1440,770&w=320&h=171&fit=min&auto=format)
Einstein's objection to quantum mechanics, where he famously said, 'God does not play dice,' mirrors the shift we see in the software world with AI.
Akin to how quantum mechanics upended determinism and introduced a probabilistic view, the emergence of Gen AI has not only introduced a paradigm of probabilistic software but has also reimagined traditional technology stacks. The early successes of Gen AI software in areas such as customer support, code generation, and photo/video editing are promising signs that we are on the path to a world with significantly enhanced software, all made possible by embracing AI's non-determinism. This probabilistic approach allows for flexibility and creativity, enabling a generation of diverse and contextually relevant applications. However, evaluating the quality of their outputs at scale is hard!
Unlike deterministic systems, which produce the same output given the same input, probabilistic models can produce different outputs for the same input on different occasions. This inherent uncertainty and variability is what prohibits Enterprises from truly leveraging Generative AI.
Challenges in Gen AI Adoption in Enterprises
With nearly one in three enterprises either using or experimenting with generative AI in at least one business function, the importance of developing and deploying LLMs is growing rapidly. As the demand for AI-driven products surges, full-stack developers, now increasingly referred to as ‘AI engineers,’ are pressured to integrate generative AI capabilities into their applications. This new paradigm is unfamiliar territory for many, and managing probabilistic LLMs presents unique challenges throughout the development lifecycle and poses significant risk while taking applications to production.
We identify four primary barriers to widespread AI adoption:
- Limited interpretability, reliability, and control.
- Exposure to new security vulnerabilities, along with complex root cause analysis and remediation.
- Technological complexity and integration challenges.
- High costs associated with development, inference, and maintenance, along with uncertainties about ROI.
At first glance, these issues may seem unrelated, but a closer look reveals that 'evaluation' is the linchpin in solving the above problems. Effective evaluation acts as a guide to test, refine, and better understand AI technologies. By establishing evaluation metrics linked to business outcomes, companies can better gauge the reliability and performance of their AI initiatives. This clearer understanding of ROI strengthens the business case for deploying AI across both internal and external use cases.
Attention (to evaluation) is all you need!
The three main stages of the 'AI development life cycle are': (i) data collection, (ii) model development, and (iii) model deployment. However, in practice, AI development is an iterative process. Post-training, engineers evaluate the AI's performance on data the model wasn't trained on. Based on the performance on the testing data, AI engineers decide to update the training dataset or the model architecture. Similarly, post-deployment, AI engineers continuously evaluate the outputs and update the training/testing dataset to be representative of usage patterns. Balancing trade-offs and identifying evaluation metrics correlated with business use cases is tedious. Hence, aiding AI engineers in the development of a testing framework by identifying a cohort of evaluation metrics that track alignment across all axes of behavior and quality is a massive value unlock.
The necessity of testing frameworks for LLMs cannot be overstated. They are fundamental to ensuring the reliability, ethical integrity, and safety of AI models, which in turn builds user trust and accelerates AI adoption. By acting as an essential layer between model infrastructure and applications, testing and evaluation frameworks enable the scalable and responsible deployment of AI technologies. As we continue to push the boundaries of what LLMs can achieve, investing in robust testing and evaluation processes will be key to unlocking their full potential and driving the next wave of AI innovation.
Enter Maxim AI
Maxim AI is strategically helping solve this problem of taking AI applications from prototype to production. A full-stack LLMOps platform that spans the entire development lifecycle, from ideation to deployment.
Our conversations with the Google - Postman duo Vaibhavi and Akshay revealed their obsession to tackle this big bold problem and doing so while keeping the Developer Experience at its core. Their attention to detail to each part of the workflow to provide delight to the AI engineer is unparalleled.
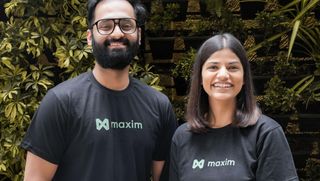
Building products at the Assistant NLP and Developer Platform teams at Google, Vaibhavi has seen this exact problem first hand and understands the implications of solving this at scale. Akshay has been solving deep developer problems for more than a decade at companies like Postman, Slack, Media.net etc. A geek at heart, he built the first commercial software at the age of 14 and has been in love with technology ever since.
Maxim has been designed from the ground up with the 'AI engineer’ persona in mind. The theme of building for a new persona played out in the cloud era, with the then-called 'cloud engineers' becoming the modern SRE/DevOps engineers. We believe that an early identification of a new developer persona in an era of a paradigm shift in technology is a winning strategy. Without a robust testing framework, it is very difficult for AI engineers to make correct decisions while experimenting. With the size of models ballooning and the cost of iteration very high, providing developers with the tools to craft their compass is a strong value proposition.
Testing cuts across the entire AI development lifecycle, necessitating a full stack testing framework such as Maxim. Maxim continuously updates golden testing datasets based on user feedback, augmenting it through synthetic data generation to ensure that the testing datasets represent current usage patterns and reflect business alignment. To enable a more collaborative and decentralized development experience, Maxim has enabled a suite of collaborative features such as prompt versioning, production logging, dashboarding, and integration into CI/CD pipelines.
By directly tackling AI development and adoption challenges, Maxim provides a comprehensive solution that simplifies the AI development process, enabling AI engineers to supercharge their applications with the power of generative AI.
Looking ahead, we believe that the advancements in multimodal AI and the maturity of the open-source ecosystem are creating a perfect storm for large-scale disruption. By solving for testing, a major hurdle in the development and deployment of AI applications, Maxim is poised to be the inflection point in the AI adoption curve.
We are very excited to partner with Maxim by leading the company's Seed Round. Our vision is for Maxim to be the go-to testing framework for generative AI applications, and we couldn't be more excited to partner with Vaibhavi and Akshay on their journey!
Written by Poorvi Vijay, Aadith Ramesh, Shambhav Tewari
Related
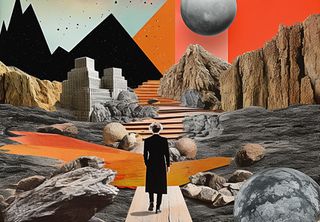
The State of GenAI in Consumer Applications
The State of GenAI in Consumer Applications
24.07.2024
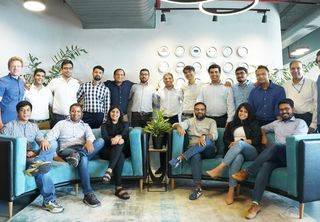
Our New Fund: All In on India
Elevation Capital announces Fund VIII of $670 million, to accelerate partnering with category defining early stage companies in India
07.04.2022
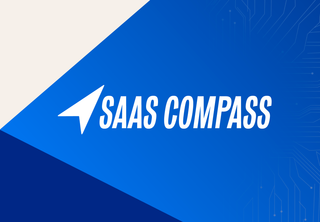
Introducing Saas Compass By Elevation
A program to supercharge global aspirations of Indian SaaS founders
11.08.2021